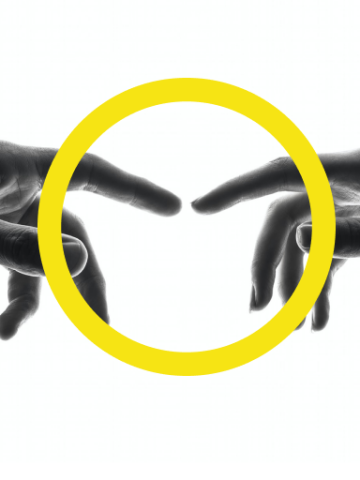
Responsible AI
February 3, 2022
Sngular + Eticas
We are joining forces with Eticas and applying a new work methodology, turning us into the first provider of ethical and trustworthy Artificial Intelligence solutions.
We interviewed Eticas’ CEO and founder Gemma Galdón to delve into the world of algorithmic auditing.
With the aim of offering our clients the highest possible value, we are constantly seeking the best technological solutions — ones that unleash the transformation and disruption processes posed to reinvent sectors across the board. The concepts of growth and integration have been part of Sngular’s DNA since it was founded and have solidified our position as a continuous technology talent connector. Meeting exceptional people who share our philosophy but bring new skills, perspectives and challenges to the table drives us forward. That’s why we couldn’t imagine better news than announcing our pioneering new alliance with Eticas.
In a moment defined by growing concern and regulation around the responsible use of technology, we are partnering with Eticas to help companies that use or plan to use AI in their business processes to create strategies, understand the risks of their algorithms and identify vulnerabilities.
With this agreement, we are also articulating the first global proposal for the integrated development of ethical, responsible and legal AI systems.
“The joint methodology that we are proposing will facilitate not only complying with the law when developing projects but also accelerating and solidifying the step from proof of concept to production. Clients will be able to work with experts in both technical and legal development,” said Nerea Luis, Sngular’s Artificial Intelligence Lead.
Nerea Luis, Artificial Intelligence Lead at Sngular, and Gemma Galdón, CEO & Founder of Eticas.
What is this joint project about?
As the discussion over a future European Union law to regulate AI points out, the health, employment, education, security, social services and justice sectors are particularly sensitive to the responsible use of artificial intelligence. Indeed, these areas are defined as “high-risk” when it comes to AI. What all of the sectors have in common is minimal tolerance for errors in algorithms. This implies the need for highly specialized teams alongside effective governance and data protection models to minimize legal, reputation and ethical risks.
Well aware of this emerging problem, at Sngular, we have built a partnership with Eticas — a pioneer in algorithmic auditing — to forge an approach that helps organizations apply joint and agile work methodologies to third-party technical projects. This will involve developing, supervising, evaluating and auditing the algorithms of data systems in high-risk contexts, where decisions will impact people directly or indirectly.
Download the range of services
What is Eticas?
Eticas is a pioneer and global leader in algorithmic auditing and ethics solutions applied to AI. It has worked in the public and private sectors, as well as with institutional actors like the UN, OECD and European Commission.
It teams up with organizations to identify algorithmic vulnerabilities and retrains AI-powered technology with better data and content. The company empowers its clients with more cognitively diverse algorithms that unearth more accurate outputs that can be turned into competitive advantages.
We spoke with Eticas’ CEO and founder Gemma Galdón to delve into the world of algorithmic auditing and gain a deeper understanding of how to guarantee the security of AI.
What drove you to create Eticas?
I created Eticas when I realized the way we approach technology often leads to undesirable social impacts, consequences and externalities. The breakneck speed of technological development often makes innovators overlook the importance of understanding and mitigating risks and negative outcomes to maximize an innovation’s positive aspects.
This compelled me to work in technology from the point of view of improving technological developments to ensure they protect people.
Could you explain the concept of ethical AI?
Ethical artificial intelligence is like healthy food, responsible consumption or cars with breaks. I believe it is the only possible AI and the only technological innovation that deserves to be called an innovation and needs to be developed. Some technology is simply undesirable, like a family sedan that can travel 300km/hour. For me, the best technology is developed with ethics and social impacts in mind.
What’s tough about technological innovation is understanding the limits of what you’re doing. Creating something that doesn’t comply with the law and harms third parties is easy. Understanding what you cannot do legally or in a socially acceptable way is hard. That’s why social concerns, legal frameworks and transparency must be built directly into technology and how it operates.
Ethical AI protects people. It is transparent and takes responsibility for its impacts. All of these worries and concerns are addressed within its code. At Eticas, we translate specific social concerns into the bones of AI or other technology.
How do you define algorithmic auditing?
Algorithmic auditing is a process that allows us to externally validate, as an independent actor, whether an algorithm is good or bad and if it’s having any impacts on certain groups. For example, algorithmic systems have traditionally discriminated against women because AI is fed historical data.
Look at the banking sector. Since women were historically not the economic heads of their households, women are underrepresented in the training databases for banking algorithms. When these data are taken without an understanding of the historic bias, the algorithms go on to reproduce the same dynamic. In this example, the algorithm ends up assigning more risk to female clients while men continue to be overrepresented in the bank’s pool of clients.
When we perform algorithmic auditing, we analyze the algorithm’s entire cycle — from the choice of training database to the implementation of the algorithmic system. We have as many as 20 identification points for biases and inefficiencies, and we test each of these 20 points to ensure that harmful or incorrect information is not perpetuated. When we find these inefficiencies, we fix them. We intervene (either through policies or technical specifications) by incorporating synthetic data and rules within the algorithms to get rid of the dysfunctions, biases and inefficiencies.
In your opinion, what are the greatest challenges for public institutions in terms of technological ethics?
The public sector has a lot of challenges when it comes to incorporating more technology, and so does society as a whole. We are living through an anomalous moment in which people — both politicians and regular citizens — speak about technology in a way that makes it sound like science fiction. We don’t have a great understanding of the current technological reality.
One of the keys for public institutions is to purchase technology wisely. But if they don’t understand technology well, it’s pretty unlikely that they will do so. At Eticas, we help public institutions buy better technology, negotiate and better understand what it can offer from a realistic perspective — not in terms of hypotheticals or “promises.”
In my opinion, the four greatest challenges for the public sector are:
- Adopting better processes for bidding and public tenders related to new technologies.
- Having internal staff that can take this on, people who know understand the technology and know how to manage bidding processes. This can be difficult because it requires people with novel professional profiles and they are taking a long time to make their way into the public sector.
- Protect citizens’ data. When a public entity buys an AI system, it needs to feed the tool with personal data. If the bidding was improper, they have poor control over the system or they don’t really understand how it even works, data becomes vulnerable.
- Lead. Public institutions need to be more than consumers of technology. They should become spaces at the forefront of the development of ethical and responsible tech. We expect the public sector to cater to a logic of service and care rather than just profit.
Those are the public sector’s four big challenges. But I’m afraid that there are still a lot of people who aren’t aware of them. We are still in a phase of defining how public institutions can fit into a healthy environment of technological development.
What characteristics should be taken into account when developing and training algorithms?
To develop and implement an algorithm, the first thing is to know is the problem you are trying to solve. So often in technology, we find solutions then search for problems instead of doing it the other way around. First and foremost, we need to understand the most pressing problems and then consider what technologies will help us solve them.
Finding the exact problem to solve is a challenge in and of itself. Then, when we identify the problem, we have to understand the data points that can help us solve it. Here’s an example: an algorithm for hospital prioritization was trained with financial data because it was trained by an insurance company. It’s a common mistake to use the data that is on hand instead of the data that you actually need. So, what can we do? Gain a clear understanding of which data will allow us to make good decisions and choose the right inputs.
After choosing the right data, another major challenge is figuring out if the data contain historical biases. That could mean checking to see if groups like women, men, people of color, children, the elderly, people from certain geographic regions, etc. are underrepresented. The layers of discrimination that we can find within algorithms can run deep. Identifying them is the key to mitigating their impacts. For instance, if we know our database includes historic information that could trigger the algorithm to make unjust decisions for certain profiles, we have mechanisms to fix it. But if we don’t identify the biases, these problems will not be solved and the biases will continue to replicate.
The risks of choices and historic data aren’t the only ones. There are also technical risks when it comes to ensuring that the databases we choose work well. For instance, we can be sure that we chose everything correctly and that the data are not historically biased, but in the model, we’ve introduced a rule that leads to new biases, discriminatory practices or deficiencies. We must be constantly monitoring how the algorithm is working to make sure that it is making good decisions.
Finally, we must assure that the implementation of algorithmic decisions is transparent. In other words, we don’t stop political technology once we’ve incorporated the algorithm. Instead, we can see who is reading the algorithmic decisions and how they are being implemented in the real world. This implementation phase is another of the key points where we can identify dysfunctions that ultimately lead to poor algorithmic decisions.
Who is responsible for algorithmic errors? The organizations using the algorithms or the technology providers?
Responsibility is one of the most important aspects in this area. That’s because it’s not always clear for those involved. For example, there’s a US case where an autonomous car hit a woman and killed her. It was discovered that the autonomous driving system was poorly designed, as it wasn’t taught to identify pedestrians when they were off the sidewalk. That is clearly a flaw since the algorithm ignored key aspects of reality and driving. However, in the contract that was signed between the driver and company, it was made patently clear that it was the driver’s responsibility. And in the end, it was determined to be the driver’s fault.
Legally, responsibility is determined by contracts. What we are witnessing is that on numerous occasions, public institutions or big companies buy technology from third parties without ensuring that they are protected and without identifying the potential impacts that could derive from its use. These cases are usually due to a lack of understanding.
If in the contract, the developer says that it's the client’s responsibility and the client signs the agreement, no matter how much we say that there is a problem with the technology, if it leads to disaster, the liability is undeniably on the consumer. At Eticas, we help our clients go over these contracts to ensure that they are protected and that the responsibility is distributed fairly. Obviously, if a client wasn’t involved in codifying an algorithm, it seems absurd to blame them for errors in a phase where they didn’t have any control. But right now, that subject is still up in the air.
Are businesses today ready to identify these kinds of errors and mitigate risks?
In our experience, neither businesses nor public institutions are ready to identify the risks. That’s because, in recent years, it’s been assumed that only engineers can develop AI technology. And this can be true in low-risk and low-impact AI use cases like sales or if the Netflix algorithm fails when recommending the next movie.
But right now, AI is making the leap from low impact to high impact, when it’s employed in spaces like health, education, security, criminal justice (like the algorithms that recommend sentences in the US), or in cases like prioritizing hospital patients, which can literally mean the difference between who lives and who dies, the impact is not comparable to a Netflix recommendation. In these cases, we have found that the input of engineers alone simply isn’t enough.
It’s like telling a team of plumbers to build an entire building. Although their skills are related, They are likely lacking experience when it comes to coordinating the massive project and making decisions with other professionals like contractors, architects and designers. It’s the same for high-impact AI. Engineers are key, but they are just one part of the process of building highly complex sociotechnical systems.
We believe that companies lack the capacity to identify risks in algorithms because their technical teams usually include engineers who, although skilled, aren’t trained to understand the details of certain areas. Most engineers don’t know how a medical algorithm works, for example. That’s why the development team has to include a doctor who is there to ensure that the algorithm responds to the concerns of the medical sector.
At Eticas, we train engineers with the knowledge that they’re lacking. We have an external sociotechnical team that changes according to market demands but is always focused on helping to make technological development in both the private and public sectors more ethical and robust.
What does this alliance with Sngular mean to Eticas?
The alliance with Sngular brings our vocation to shifting how we create technology a step forward. It makes us the first integral provider of ethical AI solutions and is key for bridging the gap between legal and ethical principles and concrete implementation.
We believe that in the near term, high-risk AI systems for areas like social services, education, health and criminal justice cannot be done with an AI that isn’t responsible and ethical by default. And we want to demonstrate how to do this in the real world. There are currently tons of people who want to know how we can take this leap forward, how we can move from principles to practice.
What we’re doing with Sngular is creating this alliance to improve the knowledge of AI from all perspectives. This includes technical perspectives as well as a better understanding of social and sociotechnical aspects that have impacts on the definition and deployment of AI. This turns us into a pioneering space of change where we will co-create experiences and concrete examples of how to develop better and higher quality AI than what’s been done up to this point.
We are taking this awesome qualitative leap in how we think, code and develop AI. But we knew we could only do it alongside a similarly pioneering organization that is also young and dynamic. That’s why we feel Sngular is the ideal partner.
Our latest news
Interested in learning more about how we are constantly adapting to the new digital frontier?
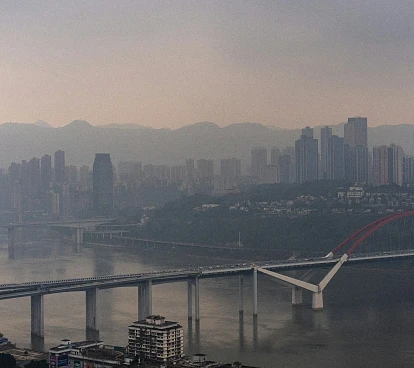
Insight
August 27, 2024
What do we do about the pollution problem?
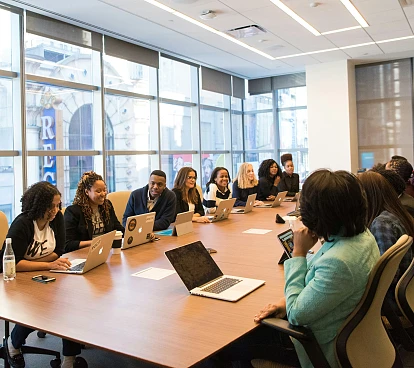
Insight
August 20, 2024
Diversity and Agility as keys to improve your organization
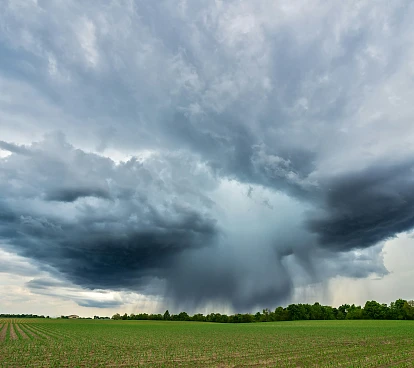
Interview
August 13, 2024
Learning more about Geoengineering with Emilio Rey